NVIDIA Gives Xavier Status Update & Announces TensorRT 3 at GTC China 2017 Keynote
by Nate Oh on September 26, 2017 10:00 AM EST- Posted in
- SoCs
- NVIDIA
- Volta
- Neural Networks
- Xavier
- GTC 2017
- Deep Learning
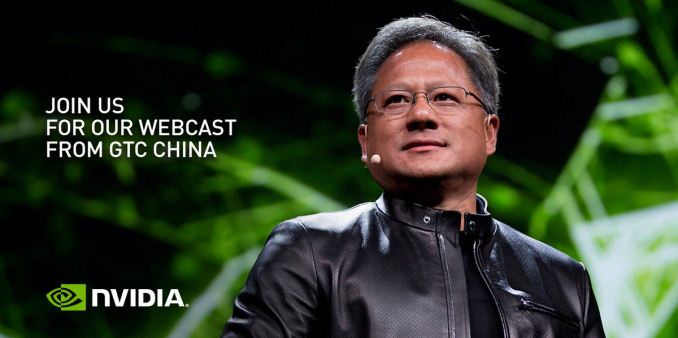
Earlier today at a keynote presentation for their GPU Technology Conference (GTC) China 2017, NVIDIA’s CEO Jen-Hsun Huang disclosed a few updated details of the upcoming Xavier ARM SoC. Xavier, as you may or may not recall with NVIDIA current codename bingo, is the company's next-generation SoC.
Essentially the successor to the Tegra family, Xavier is planned to serve several markets. Chief among these is of course automotive, where NVIDIA has seen increasing success as of late. However, similar to their talk at the primary GTC 2017, at GTC China NVIDIA is pitching Xavier as an “autonomous machine processor,” identifying markets beyond automotive such as drones and industrial robots, pushing a concept in line with NVIDIA’s Jetson endeavors. As a Volta-based successor to the Pascal-based Parker, Xavier does include Volta’s Tensor cores, something that we noted earlier this year, and is thus more suitable than previous Tegras for the deep learning requirements in autonomous machines.
In the keynote, Jen-Hsun additionally revealed updated sampling dates for the new SoC, stating that Xavier would begin in Q1 2018 for select early development partners and Q3/Q4 2018 for the second wave of partners. This timeline actually represents a delay from the originally announced Q4 2017 sampling schedule, and in turn suggests that volume shipments are likely to be in 2019 rather than 2018.
Meanwhile on the software side of matters, Jen-Hsun also announced TensorRT 3, with a release candidate version immediately available as a free download for NVIDIA developer program members. Introduced under its current branding in 2016 and a critical part of NVIDIA's neural networking software stack, TensorRT is programmable AI inference software that takes computational graphs created by traditional frameworks (e.g. Caffe, TensorFlow) and then compiles and optimizes it for NVIDIA CUDA hardware. At the moment, this includes the Tesla P4, Jetson TX2, Drive PX 2, NVIDIA DLA, and Tesla V100. During the keynote, NVIDIA also formally disclosed that a number of large Chinese companies (Alibaba, Baidu, Tencent, JD.com, and Hikvision) were now utilizing TensorRT.
Source: NVIDIA
10 Comments
View All Comments
Gc - Tuesday, September 26, 2017 - link
"deep learning requirements in autonomous machines""Learning" requirements? Or "inference/recognition" requirements?
Some safety critical devices, like medical devices, are licensed based on behavior during licensing tests. Any software change requires expensive retesting, so no updates. The most advertised pattern recognition capabilities of future cars and drones regard self-driving, which means those are safety critical capabilities. Testing them to be sure they cannot learn behavior outside the licensed safety requirements, no matter what driving conditions they experience over years of use, would seem to make model testing even more complex. Are self-driving controls, that learn, licensed now?
Rather than only testing the model design once for all cars of that model, another approach is to test every car at its annual inspection. The test might take long to do physically, so someone might propose disconnecting the control unit and testing virtually, perhaps with accelerated time. That adds additional risks (disconnectable controls may become less reliable, changeable clock may not reset correctly).
If learning is enabled, then training seems a little like dreaming, rehearsing situations remembered or imagined variations. When would it dream, at stop lights? If it is an important dream lesson from a near miss that just occured, or a wheel that is getting out of balance, it might not 'want' to wake up and drive before it learned the lesson.
Fallen Kell - Wednesday, September 27, 2017 - link
I think you misunderstand deep learning. There are multiple parts to deep learning, first of which is the construction and design of the neural network itself (i.e. how many layers it should have, how many inputs and outputs, etc., etc.). Then there is the training of the network. This is the part where the actual "learning" occurs. Then finally there is the operation of the already trained network. This final stage is the part that Nvidia is targeting with Xavier. There is no more actual learning occurring, just the implementation of the already learned behaviors.MrSpadge - Wednesday, September 27, 2017 - link
I think he understands this well. That's why he asked in the first place whether "Learning" requirements?" actually meant "inference/recognition requirements". It seems rather obvious they mean the latter, as the former would lead to all kinds of problems he mentioned.Fallen Kell - Wednesday, September 27, 2017 - link
Except that it isn't "inference/recognition" in deep learning, it is simply a complex probability function, based on the training data typically with risk/reward functions taken into account (for instance with driving, the network is probably rewarded for getting to the destination in a timely fashion, while obeying various driving rules/laws, and at the same time keeping the passengers safe, as well as other humans safe without damage to the vehicle or other property). It is unclear if higher weights are placed on the passenger, or other humans, or to the vehicle or other vehicles, that all depends on the reward set.As the network is trained, weights are adjusted between the various nodes in the network, and in the case of something like driving, previous states are also part of the input (as the algorithm would need to remember what it just did and why, to take those previous actions into account for the next action to take). Once the weights have been defined on the links between the various nodes and layers of the network, using that network is just a simply matter of running through the probabilities to generate the highest probable action/decision that received the highest reward from during the training phases.
Inference/recognition makes an assumption that a pattern is being followed, when in reality, it is just a probability function.
skavi - Tuesday, September 26, 2017 - link
I wish they would come back to mobile. We need more competition and custom (non ARM) designs in the Android space.milkod2001 - Wednesday, September 27, 2017 - link
NV likes fat margins the same as Intel or Apple. I don't see NV entering Android space anytime soon.The place is already overcrowded.Santoval - Wednesday, September 27, 2017 - link
I assume by "non ARM" you mean "custom or semi-custom CPUs with ARM ISA", right? Currently there are only two options for ISA of mobile CPUs : ARM and MIPS. The latter currently undergoes a process of either restructuring or shattering, x86 is out of the mobile game, so what remains is only ARM.lada - Friday, September 29, 2017 - link
and RISC-V , which is free and open. And very efficient, as much that nVidia chose it to be the ISA for the GPUs' control MCUs. But it has a great potential to be the main CPU's ISA (32bit, 64bit and 128bit ISAs are specced).tipoo - Wednesday, September 27, 2017 - link
Yeah, Denver 1 was one of the few designs that went as wide as Apple. Shame about its binary translator choking up on anything harder to predict. Denver 2 with that shortcoming addressed may have been interesting.